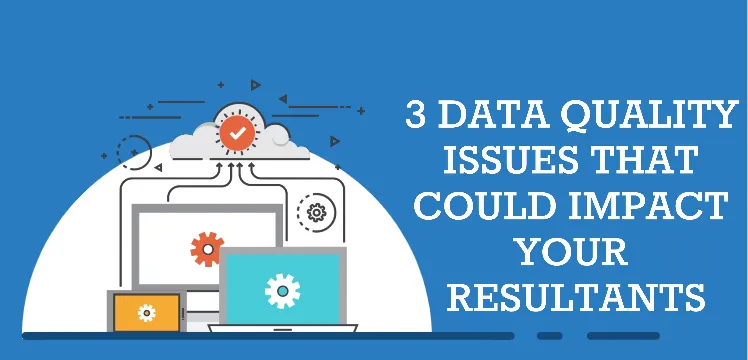
Google"big information," and autocomplete for your research tells quite the narrative:"Big information is your long run.
These are a few fascinating announcements, but what is often missing in the dialog about large information is the high price of poor data.
If your business prides itself making data-driven conclusions , it is important to realize that those choices will probably only be as good as your own information. Poor data quality costs the U.S. market $3.1 trillion annually , and it is developing a crisis of religion across several businesses.
As per a recent Gartner report, over fifty percent of senior advertising leaders have been disappointed in the results they have observed from investments in data analytics. Because of this, only 54 percent of the actions are affected by data.
The significance of quality information can't be overstated, but frequently leaders do not understand where their information collection and analysis is still breaking down. Listed below are 3 data-quality problems you May Not Know about.
1. Data models break down with volume
As unmanaged outliers can skew information as time passes, many data units start to break down as the quantity of information increases. This does not indicate that data units abruptly quit functioning. Most information quality problems within a company exist in the beginning, but they do not become evident until they achieve a certain degree.
This is very relevant today, as stay-at-home orders have induced retail information to dry up immediately . When constraints are lifted, it is unlikely that customer behaviour will be just as it had been earlier. Clients could be spending ordering more products on the web. Many businesses find that their older information is no more relevant.
Nearly all businesses will likely be re-scaling their information in the next several years. They will need to upgrade their versions to account for changes in customer behaviour.
2. Different departments use the same data for different purposes
Nowadays, organizations are generating an increasing number of data, which information is used to notify company decisions at each level. Key Performance Indicators (KPIs) generated by an ecommerce group could possibly be employed by the advertising division to target a brand new customer segment. Or, exactly the same information may be utilized at the greatest levels to construct models around fiscal performance to notify hiring decisions.
The problem is that the people creating that information normally don't have any idea who's using it or how it's used. It is unclear who is responsible for the accuracy and management of the information. This becomes problematic if it is used to induce conclusion.
3. Anomalies become harder to manage as data balloons
That does not necessarily mean your information is not true, but outliers (like seasonal changes ) has to be accounted for.
If you possess an apparel business that sees a massive requirement for red sweaters from the lead-up to Christmas, then you are able to readily recognize the origin and manage it appropriately. But fully removing your outliers isn't the answer, as some sections might require this outlier data, like your own procurement and merchandising teams.
This makes more complex as your organization begins collecting and using additional information. Every brand new metric will possess its own trends and anomalies, and also you can not manually research and fix each of these outliers. Since Credera's Chief Data Officer Vincent Yates stated at a blog article,"Classifying anomalies in data is an artwork, and practically no firm has a mechanism to codify those annotations globally."
As time passes, the issue with incorrect data sets generates a snowball effect. This information can not be used to predict future demand together with any precision, which erodes organizational confidence in data.