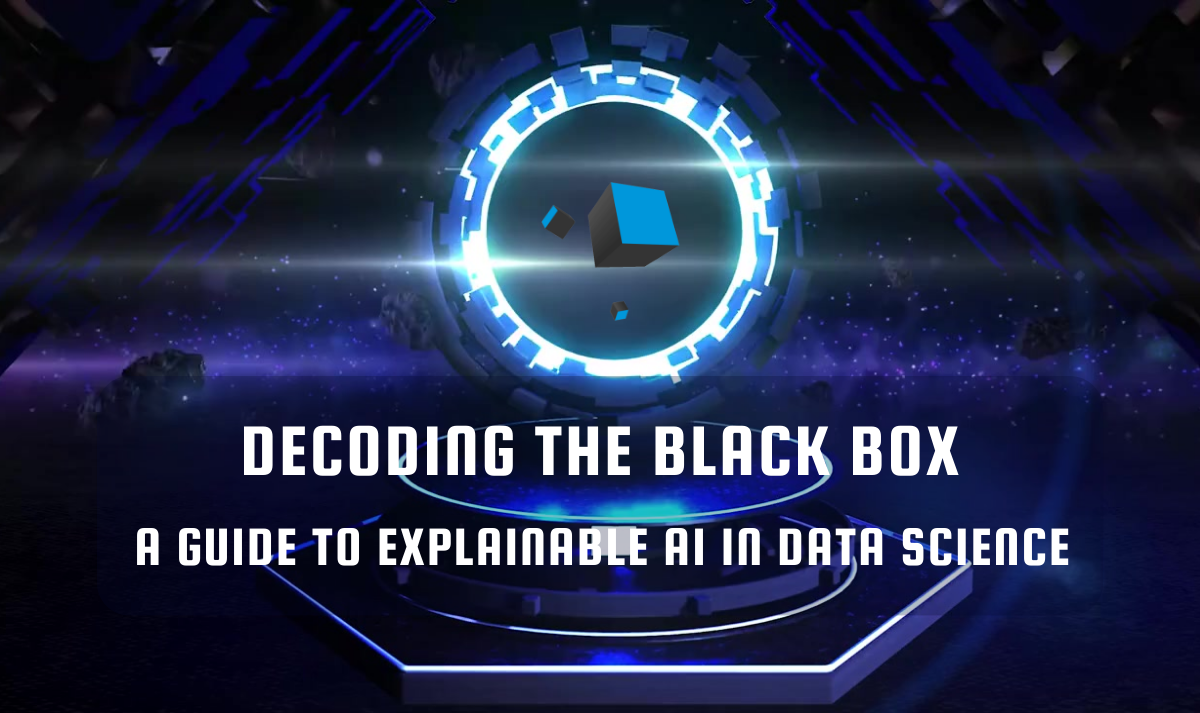
Artificial Intelligence (AI) has become an integral part of our lives, powering systems that impact our decisions in healthcare, finance, and even criminal justice. However, the opaqueness of complex machine learning models, often referred to as "black boxes," has raised concerns about transparency, accountability, and ethics. In this comprehensive guide, we will delve into the world of Explainable AI (XAI), exploring the importance of understanding these black boxes and the techniques that can help decode them.
Understanding the Black Box
At its core, a black box in AI refers to a model whose internal workings are not easily interpretable by humans. This lack of transparency can lead to challenges in understanding how and why a model arrives at a specific decision. Machine learning models, particularly deep neural networks, are notorious for their intricate structures, making it difficult to trace the logic behind their outputs.
The opacity of black box models poses significant challenges, especially when these models are deployed in critical domains where decisions impact individuals' lives. To address these challenges, the concept of Explainable AI has emerged as a crucial area of research and development.
The Need for Explainability
-
Trust and Accountability:
- In applications such as healthcare and finance, where AI plays a pivotal role in decision-making, establishing trust is paramount.
- Explainability helps in holding AI systems accountable for their actions, providing insights into the decision-making process and fostering trust among users.
-
Ethical Considerations:
- The inherent biases present in data can propagate into AI models, leading to discriminatory outcomes.
- Explainable AI allows practitioners to identify and rectify biases, promoting fairness and ethical use of AI technologies.
-
Regulatory Landscape:
- With the increasing influence of AI in various industries, regulations and standards are emerging to ensure transparency and accountability.
- Compliance with these regulations often requires organizations to provide explanations for automated decisions, making Explainable AI not just desirable but a legal requirement in certain domains.
Techniques for Explainable AI
-
Feature Importance and Visualization:
- Analyzing which features contribute the most to model decisions provides valuable insights.
- Visualization tools help in presenting these insights in a comprehensible manner, making it easier for stakeholders to grasp the driving factors behind decisions.
-
Local and Global Explanations:
- Understanding how a model arrives at a specific decision for an individual instance (local explanation) versus gaining insights into the overall model behavior (global explanation) are essential aspects of XAI.
- Local explanations provide context for specific predictions, while global explanations offer a broader understanding of the model's functioning.
-
Surrogate Models:
- Building simpler, interpretable models that approximate the behavior of complex models helps bridge the gap between transparency and accuracy.
- Surrogate models act as proxies for black box models, offering a more understandable representation of the decision-making process.
-
LIME (Local Interpretable Model-agnostic Explanations):
- LIME is a technique that generates locally faithful explanations for black-box models.
- It allows users to understand the decision rationale for specific instances by approximating the black box's behavior in the local vicinity of the instance.
Real-world Applications
Explainable AI has far-reaching applications across various industries:
-
Healthcare:
- Understanding the basis of a diagnostic recommendation made by an AI system is crucial for doctors and patients alike.
- Explainability in healthcare AI ensures that medical professionals can trust and comprehend the decisions made by AI models, ultimately leading to improved patient care.
-
Finance:
- Addressing bias and promoting fairness in financial decisions is a critical aspect of AI applications in finance.
- Regulatory compliance in the financial sector requires institutions to provide explanations for automated decisions, making Explainable AI an essential component of responsible financial AI.
-
Criminal Justice:
- In the criminal justice system, the role of explainability is crucial in ensuring fair sentencing.
- Understanding how AI models arrive at decisions related to legal matters helps in avoiding unjust outcomes and upholding the principles of justice.
Challenges and Future Directions
-
Balancing Accuracy and Interpretability:
- One of the primary challenges in Explainable AI is finding the right balance between the accuracy of predictions and the interpretability of models.
- Striking this balance is crucial, as overly complex models might achieve high accuracy but at the cost of transparency.
-
Novel Techniques:
- Researchers are exploring novel techniques to enhance the explainability of AI models.
- Integrating interpretability into the model training process is an area of active research, aiming to produce models that are inherently more transparent.
-
Ethical Considerations in XAI Research:
- As the field of Explainable AI progresses, ethical considerations become increasingly important.
- Ensuring fairness, avoiding unintended consequences, and promoting ethical practices in XAI research are essential for responsible AI development.
Conclusion
As AI continues to permeate various aspects of our lives, ensuring transparency and understanding in model decisions becomes paramount. Explainable AI serves as a guiding light in navigating the complexities of the black box, fostering trust, accountability, and ethical use of AI technologies. Embracing explainability is not just a choice; it's a necessity for the responsible deployment of artificial intelligence in our increasingly interconnected world. As practitioners, researchers, and policymakers in the AI community, it is our collective responsibility to champion the cause of Explainable AI, ensuring that the benefits of AI are realized without compromising on transparency and fairness.