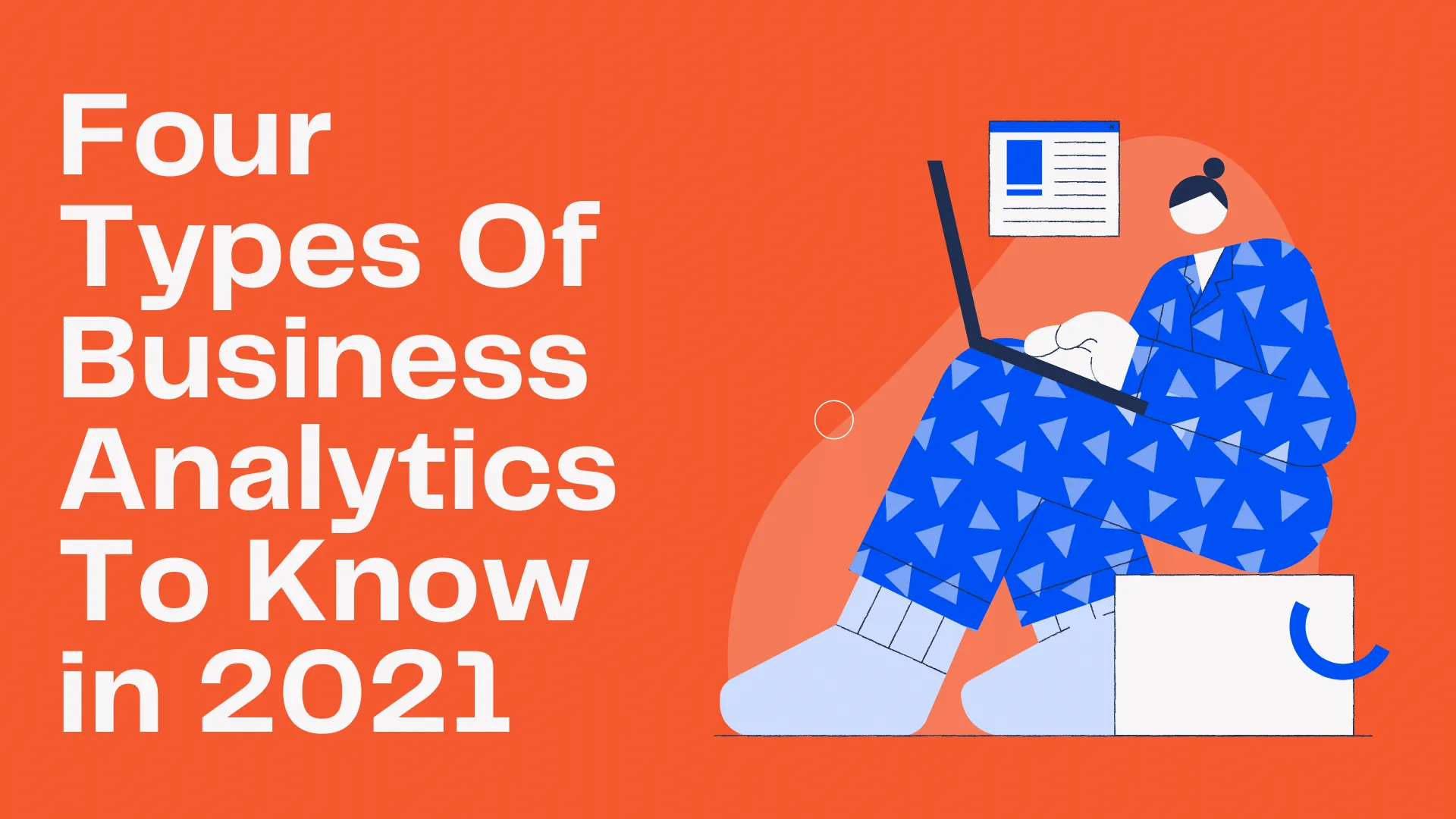
For distinct phases of business analytics enormous number of information is processed in different actions. These four kinds together answer what a business needs to understand - from what is happening in the business to what answers to be embraced for optimising the purposes.
The four kinds of analytics are often implemented in phases and no 1 kind of analytics is reportedly better than another. They're each and every one of them offers another insight. With information being significant to so many varied sectors- from manufacturing to power grids, the majority of the businesses rely upon one or each these kinds of analytics. With the Ideal choice of analytical Methods, big data can provide richer insights for your businesses.
Before diving deeper into each of these, let’s define the four types of analytics:
1) Descriptive Analytics
2) Diagnostic Analytics
3) Predictive Analytics
4) Prescriptive Analytics
Let’s understand these in a bit more depth.
1. Descriptive Analytics
This may be called the easiest type of analytics. The powerful size of data that is big is beyond human understanding and the very first stage hence entails transferring the information into comprehensible chunks. The objective of the analytics kind is simply to summarise the findings and comprehend what's happening.
One of some frequently used phrases, what people phone as complex analytics or business intelligence is essentially usage of descriptive data (arithmetic operations, mean, median, maximum, percent, etc.) on present data. It's said that 80 percent of business analytics chiefly entails descriptions based on aggregations of previous performance. It's a significant thing to produce raw information clear for shareholders, investors and managers. This way it becomes simple to spot and tackle the areas of strengths and flaws such it may assist in strategizing.
The two chief techniques involved are information aggregation and data mining saying that this technique is only used for comprehending the inherent behaviour and to not create any estimations. By mining historic data, businesses can examine the customer behaviours and engagements with their companies that could be useful in targeted advertising, service development, etc.
2. Predictive Analytics
As stated previously, predictive analytics can be used to forecast future results. But it's necessary to be aware it can't predict if an event will happen later on; it only forecasts what will be the probabilities of the occurrence of this function.
The gist of predictive analytics will be to invent models like the present information is known to extrapolate the future occurrence or just, predict the long term information. Among the typical uses of predictive analytics is found in opinion analysis where each of the remarks posted on social websites are gathered and analyzed (present text information ) to predict that the individual's opinion on a specific topic as beingpositive, neutral or negative (future forecast ).
Therefore, predictive analytics involves validation and building of models that offer accurate predictions. Predictive analytics is based on machine learning algorithms such as random woods, SVM, etc. and data for studying and analyzing the information. Normally, companies need skilled information scientists and machine learning specialists for constructing these models.
The prediction of future information relies on the present information as it can't be accessed otherwise. If the version is correctly tuned, it may be utilized to support complicated predictions in sales and promotion. This goes a step forward of their normal BI in providing accurate predictions.
3. Diagnostic Analytics
Diagnostic analytics is used to ascertain why something happened before. It's characterized by techniques like drill-down, data discovery, data mining and correlations. Diagnostic analytics requires a deeper look at data to comprehend the root causes of these events. It's useful in determining what events and factors contributed to the outcome. It mostly uses probabilities, likelihoods, and the distribution of results for the analysis.
At a time series data of earnings, diagnostic analytics would help you realize why the earnings have reduction or increase for a particular year or so. However, this sort of analytics has a limited ability to offer actionable insights. It just gives an understanding of causal relationships and sequences while looking backward.
A couple of methods that utilizes diagnostic analytics include feature significance, principle components analysis, sensitivity analysis, and conjoint analysis.
4. Prescriptive Analytics
The basis of the analytics is predictive analytics but it extends beyond the three mentioned above to indicate the future solutions. It can suggest all positive outcomes based on a predetermined course of action and suggest various course of activities for to a specific outcome. Consequently, it uses a powerful feedback system that constantly learns and updates the association between the actions and the outcome.
The computations include optimisation of several functions which are linked to the desired result. By way of instance, while calling for a taxi online, the program uses GPS to link you to the proper driver from among a range of drivers located nearby. Consequently, it optimises the space for quicker arrival time.
It makes sure if the key performance metrics are contained in the solution. The optimization version will further work on the effects of the previously made predictions. Because of its capability to suggest favorable options, prescriptive analytics is the last frontier of innovative analytics or information science, in the modern term.
Cloud Computing Courses You May Like:
One To One Azure Cloud Training