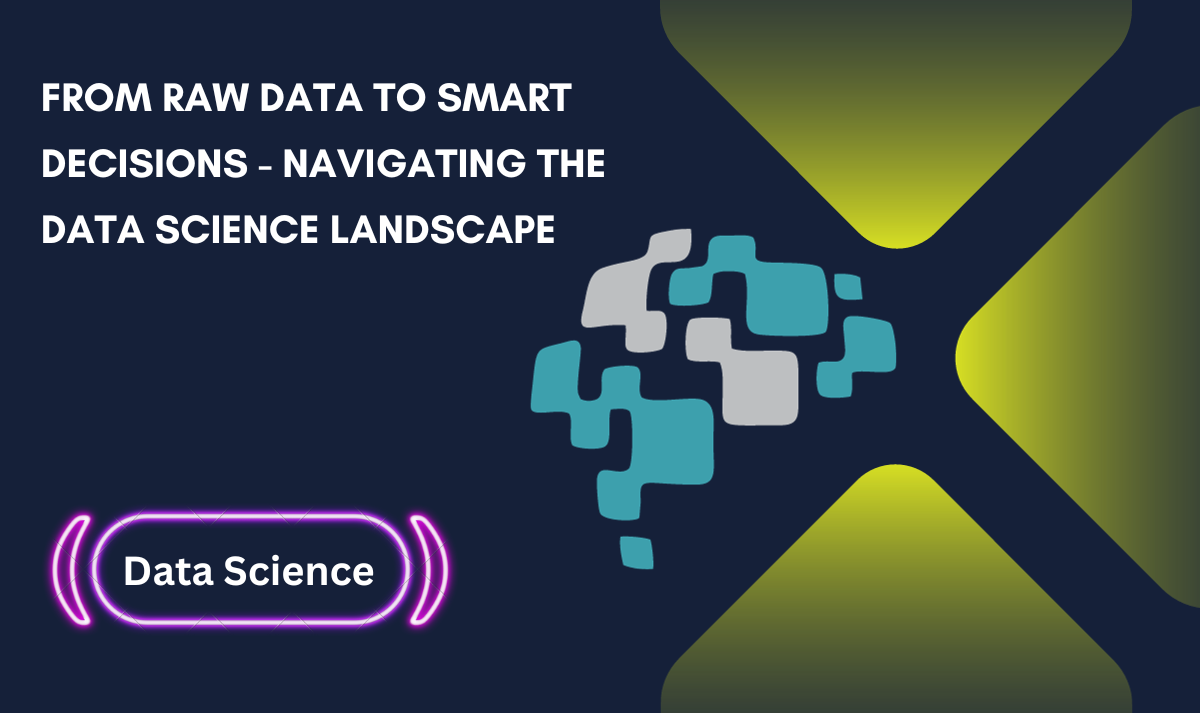
In the digital era, where information is abundant and the volume of data generated daily is staggering, businesses and individuals are faced with the challenge of making sense of raw data to derive meaningful insights. Enter data science—a multidisciplinary field that combines statistical methods, programming skills, and domain expertise to transform raw data into actionable intelligence. In this comprehensive exploration, we will embark on a journey through the data science landscape, understanding its fundamental principles, methodologies, and the transformative impact it has on decision-making.
Understanding the Essence of Data Science
Data Collection: The Genesis of the Journey
The journey from raw data to smart decisions commences with data collection. In this initial phase, data scientists gather information from diverse sources such as databases, APIs, sensor networks, and more. The challenge lies not just in accumulating data but ensuring its relevance, accuracy, and completeness. The variety of data types—numerical, textual, categorical, or multimedia—adds complexity to the collection process.
Data Cleaning and Preprocessing: Refining the Raw Material
Raw data seldom arrives in pristine condition. It is often riddled with errors, missing values, and inconsistencies. Data cleaning and preprocessing are indispensable steps where data scientists refine the raw material. This involves handling missing data, normalizing formats, and addressing outliers. The objective is to create a clean, high-quality dataset ready for analysis.
Exploratory Data Analysis (EDA): Unveiling Patterns and Trends
Once the data is prepared, the journey advances to exploratory data analysis (EDA). This phase involves visualizing and summarizing data to uncover patterns, trends, and potential relationships. Descriptive statistics, data visualizations, and correlation analyses play a crucial role in gaining initial insights and forming hypotheses about the data.
Feature Engineering: Crafting Informative Variables
Feature engineering is an art within data science, involving the selection, transformation, or creation of relevant features (variables) that contribute to the predictive power of a model. This step requires domain knowledge and creativity to ensure that the chosen features capture the essence of the data and improve the model's performance.
The Role of Machine Learning in Data Science
Supervised Learning: Predicting Outcomes
In supervised learning, algorithms are trained on a labeled dataset where the outcome variable is known. The goal is to learn the mapping between input features and the target variable, enabling the model to make predictions on new, unseen data. Common supervised learning tasks include classification and regression.
Unsupervised Learning: Discovering Hidden Patterns
Unsupervised learning deals with unlabeled data and aims to discover inherent patterns or structures within the dataset. Clustering, dimensionality reduction, and association rule mining are common unsupervised learning techniques. This approach is particularly useful when the objective is to explore the inherent structure of the data.
Model Evaluation and Selection: Assessing Performance
The effectiveness of a machine learning model is assessed through rigorous evaluation and selection processes. Metrics such as accuracy, precision, recall, and F1 score provide insights into the model's performance. Cross-validation techniques help ensure that the model generalizes well to new data.
Model Deployment: Turning Insights into Action
Once a model has proven its efficacy, it can be deployed to make predictions on new, real-world data. This transition from experimental to operational involves considerations such as scalability, real-time processing, and integration with existing systems. Deployed models contribute directly to making smart decisions based on data-driven insights.
The Impact of Data Science Across Industries
Healthcare: Enhancing Patient Outcomes
In the healthcare sector, data science is revolutionizing patient care, treatment plans, and disease prevention. Predictive modeling helps identify at-risk patients, personalized medicine tailors treatments based on individual profiles, and data-driven insights contribute to public health strategies.
Finance: Risk Management and Fraud Detection
Financial institutions leverage data science for risk management, fraud detection, and customer relationship management. Predictive analytics assess credit risk, anomaly detection algorithms identify fraudulent activities, and personalized financial recommendations enhance customer experiences.
E-commerce: Personalization and Customer Engagement
Data science powers recommendation engines in e-commerce platforms, providing personalized product suggestions based on user behavior. Customer segmentation, sentiment analysis, and dynamic pricing strategies contribute to improved customer engagement and satisfaction.
Manufacturing: Process Optimization and Quality Control
In manufacturing, data science optimizes production processes, predicts equipment failures through predictive maintenance, and enhances quality control. Real-time monitoring of manufacturing lines ensures efficiency, while predictive analytics minimizes downtime.
Marketing: Targeted Campaigns and Customer Insights
Data science plays a pivotal role in marketing by analyzing customer behavior, segmenting target audiences, and optimizing advertising campaigns. A/B testing, customer churn prediction, and sentiment analysis contribute to data-driven marketing strategies.
Challenges and Ethical Considerations in Data Science
Data Privacy and Security: Safeguarding Sensitive Information
As the collection and analysis of data become more widespread, concerns about data privacy and security intensify. Ensuring compliance with regulations, implementing robust encryption techniques, and establishing ethical guidelines for data use are paramount to maintaining public trust.
Bias in Models: Mitigating Unintended Consequences
Machine learning models are susceptible to biases present in training data, leading to potential discrimination or unfair outcomes. Addressing bias in models requires careful consideration of dataset composition, feature engineering, and ongoing monitoring to mitigate unintended consequences.
Interpretable Models: Balancing Complexity and Understandability
The increasing complexity of machine learning models, particularly deep learning models, poses challenges in interpretability. Striking a balance between model accuracy and interpretability is crucial, especially in fields where transparency and accountability are paramount.
Ethical Use of AI: Considering Societal Impact
The ethical use of artificial intelligence (AI), a subset of data science, extends beyond individual models to broader societal implications. This includes responsible deployment of AI in decision-making processes, addressing algorithmic accountability, and fostering inclusivity in AI applications.
The Future of Data Science: Emerging Trends and Innovations
Explainable AI (XAI): Enhancing Model Transparency
The demand for transparency in AI models has led to the emergence of Explainable AI (XAI). XAI focuses on developing models that provide interpretable explanations for their decisions, enabling users to understand and trust the output of complex algorithms.
Automated Machine Learning (AutoML): Streamlining Model Development
Automated Machine Learning (AutoML) aims to democratize the machine learning process by automating various stages, including feature engineering, model selection, and hyperparameter tuning. This trend is making data science more accessible to individuals with varying levels of expertise.
Edge Computing: Processing Data at the Source
Edge computing involves processing data near the source of generation rather than relying on centralized cloud servers. This approach is gaining prominence in data science, especially in applications where real-time processing is critical, such as Internet of Things (IoT) devices and autonomous systems.
Conclusion: Empowering Decision-Making Through Data Science
The journey from raw data to smart decisions is a complex and multifaceted process that demands expertise, creativity, and ethical considerations. Data science, with its amalgamation of statistical methods, machine learning algorithms, and domain expertise, has emerged as the linchpin in this transformative journey.
As we navigate the data science landscape, it becomes evident that the impact of data science extends far beyond the realm of analysis and prediction. It has become a catalyst for innovation, driving advancements in various industries and shaping the way organizations approach decision-making.
The challenges and ethical considerations inherent in data science underscore the importance of responsible and transparent practices. Striking a balance between leveraging the power of data for societal good and safeguarding individual privacy and fairness is an ongoing endeavor.
Looking ahead, the future of data science holds promise with emerging trends such as Explainable AI, Automated Machine Learning, and Edge Computing. These innovations are poised to make data science more accessible, interpretable, and efficient, paving the way for a new era of data-driven decision-making.
In essence, from raw data to smart decisions, the data science landscape is a dynamic and evolving terrain that empowers organizations and individuals to harness the full potential of information. As we continue on this journey, the synergy between technology, ethics, and human ingenuity will shape a future where data science is not just a tool but a cornerstone of informed and impactful decision-making.