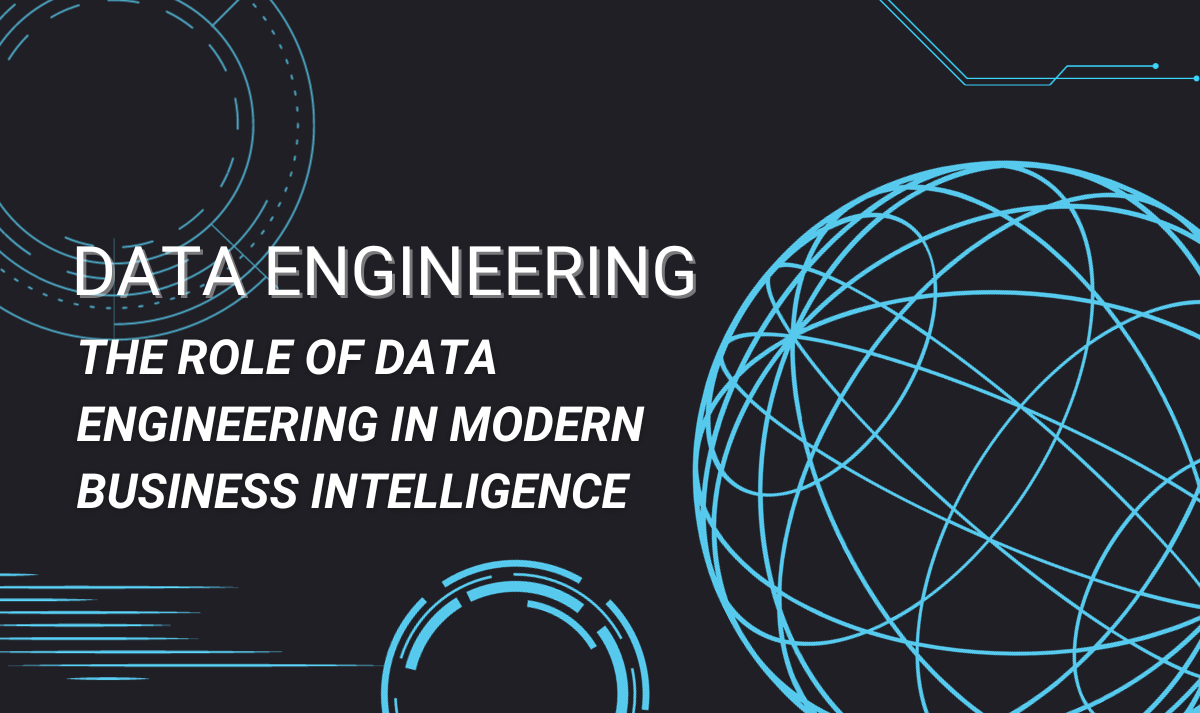
In the rapidly evolving landscape of modern business, data has become the lifeblood of organizations. The ability to harness, analyze, and derive insights from vast amounts of data is a critical factor in gaining a competitive edge. This is where the role of data engineering in modern business intelligence comes to the forefront, acting as the backbone that supports the entire data ecosystem. In this extensive exploration, we will delve into the pivotal role that data engineering plays in shaping and enhancing the landscape of business intelligence.
1. Understanding the Foundation: Data Engineering Basics
Before we explore the role of data engineering in business intelligence, it's crucial to grasp the fundamentals of data engineering. At its core, data engineering involves the design, development, and management of data architecture, infrastructure, and tools that facilitate the collection, storage, and processing of data. It lays the groundwork for creating a robust data pipeline that ensures the smooth flow of information from various sources to downstream applications.
In the context of business intelligence, data engineering provides the foundation for a scalable and efficient data infrastructure. This includes setting up databases, data warehouses, and data lakes, as well as establishing ETL (Extract, Transform, Load) processes to transform raw data into a format suitable for analysis.
2. Bridging the Gap: Data Integration and Consolidation
One of the primary roles of data engineering in business intelligence is to bridge the gap between disparate data sources. Modern organizations deal with data from a multitude of origins, such as internal databases, external APIs, cloud services, and more. Data engineering ensures seamless integration of these diverse datasets into a unified and coherent format, laying the groundwork for comprehensive analysis.
Data consolidation is a critical step in the business intelligence process. It involves bringing together data from different departments, systems, and sources, creating a centralized repository. This consolidation not only enhances data accessibility but also ensures data consistency, which is crucial for accurate and reliable business intelligence insights.
3. Enabling Scalability: Handling Big Data Challenges
As the volume of data generated by businesses continues to grow exponentially, scalability becomes a paramount concern. Data engineering addresses the challenges posed by big data, ensuring that systems can handle massive amounts of information efficiently. This involves the use of distributed computing frameworks, parallel processing, and technologies like Apache Hadoop and Apache Spark.
Scalability is crucial for modern business intelligence platforms, allowing organizations to expand their data infrastructure as their needs evolve. Data engineering provides the architecture and tools necessary to scale storage and processing capabilities, ensuring that business intelligence systems can handle the ever-increasing demands of data analysis.
4. Crafting Efficient Data Pipelines: ETL Processes
The Extract, Transform, Load (ETL) process lies at the heart of data engineering and is instrumental in shaping the data journey from source to analysis. In the context of business intelligence, ETL processes are responsible for extracting raw data from source systems, transforming it into a consistent format, and loading it into a data warehouse or data lake for analysis.
Efficient ETL processes are essential for ensuring data quality, as they involve cleaning, validating, and enriching data during the transformation phase. Data engineering teams design and optimize these pipelines to streamline the flow of information, minimizing latency and ensuring that business intelligence systems are fed with accurate and up-to-date data.
5. Data Governance and Security: Safeguarding Information Assets
In the realm of business intelligence, the importance of data governance cannot be overstated. Data engineering plays a pivotal role in establishing and enforcing data governance policies, ensuring that data is handled with integrity, confidentiality, and compliance with regulatory requirements.
Data security is a paramount concern, especially as organizations accumulate vast amounts of sensitive information. Data engineering involves implementing robust security measures, including encryption, access controls, and audit trails, to safeguard data throughout its lifecycle. This is critical for building trust in business intelligence insights and maintaining the confidentiality of strategic information.
6. Real-time Analytics: The Need for Speed
In the fast-paced business environment, the ability to derive insights in real-time has become a competitive advantage. Data engineering is at the forefront of enabling real-time analytics by designing data pipelines that support low-latency processing and analysis.
The shift towards real-time business intelligence requires data engineering teams to implement technologies like stream processing and in-memory databases. These innovations allow organizations to analyze data as it is generated, enabling quick decision-making and response to dynamic market conditions.
7. Supporting Advanced Analytics: Machine Learning and AI Integration
Business intelligence is no longer confined to retrospective reporting; it now encompasses predictive and prescriptive analytics. Data engineering facilitates the integration of advanced analytics techniques, such as machine learning and artificial intelligence, into business intelligence platforms.
Data engineering teams work on creating the infrastructure required for training machine learning models, deploying them into production environments, and integrating the insights derived from these models into business intelligence dashboards. This fusion of traditional business intelligence with advanced analytics empowers organizations to make data-driven predictions and optimize decision-making processes.
8. Democratizing Data Access: Empowering Business Users
One of the transformative aspects of modern business intelligence is the trend towards democratizing data access. Traditionally, data was siloed within IT departments, accessible only to those with technical expertise. Data engineering is instrumental in breaking down these barriers, enabling business users to access and analyze data without extensive technical knowledge.
Through the creation of user-friendly interfaces, self-service analytics tools, and intuitive dashboards, data engineering empowers business users to explore and derive insights from data independently. This democratization of data access fosters a data-driven culture within organizations, where decision-makers at all levels can leverage insights to drive innovation and efficiency.
9. Evolution of Data Warehousing: Cloud-Native Solutions
The landscape of data warehousing has undergone a significant transformation, moving from on-premises solutions to cloud-native architectures. Data engineering plays a crucial role in this evolution by architecting and migrating data warehouses to cloud platforms.
Cloud-based data warehouses offer advantages such as scalability, flexibility, and cost-effectiveness. Data engineering teams are responsible for designing and optimizing these cloud-native solutions, ensuring seamless integration with other cloud services and providing organizations with the agility needed to adapt to changing business requirements.
10. Continuous Improvement: Monitoring and Optimization
The role of data engineering in modern business intelligence extends beyond the initial setup of infrastructure and pipelines. Continuous monitoring and optimization are integral aspects of ensuring the efficiency and effectiveness of the data ecosystem.
Data engineering teams employ monitoring tools to track the performance of data pipelines, identify bottlenecks, and proactively address issues. Continuous optimization involves refining ETL processes, upgrading infrastructure, and incorporating new technologies to keep the data engineering stack aligned with evolving business needs.
Conclusion: The Synergy of Data Engineering and Business Intelligence
In the dynamic landscape of modern business, the synergy between data engineering and business intelligence is undeniable. Data engineering forms the backbone, providing the infrastructure, processes, and governance necessary for the effective harnessing of data. As organizations strive to become more data-driven, the role of data engineering in modern business intelligence will continue to evolve, shaping the way data is collected, processed, and transformed into actionable insights.
From establishing the foundations of data architecture to enabling real-time analytics and integrating advanced analytics techniques, data engineering is the linchpin that propels business intelligence into the future. As technology advances and the volume of data continues to grow, the role of data engineering will only become more critical, driving innovation and empowering organizations to thrive in the data-driven era.