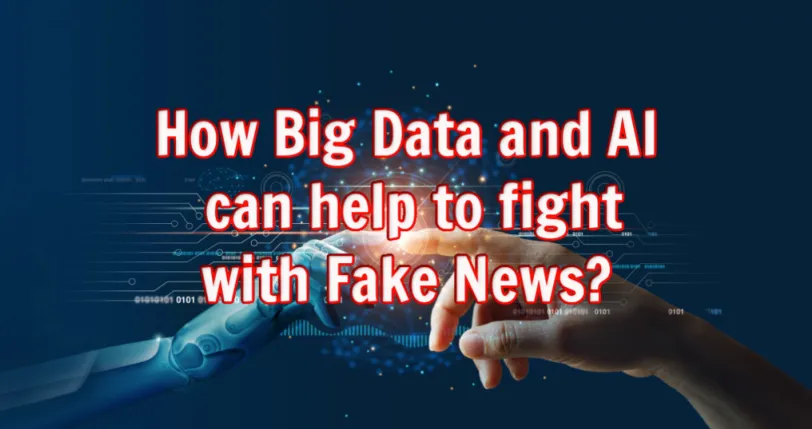
Fake news and disinformation have become a worldwide threat for data integrity and therefore are driving distrust towards individuals, governments, and communities globally. We are inundated with disinformation on a daily basis through news reports, pictures, videos, and memes.
Twisting details to further a program isn't a new issue. On the other hand, the explosive development of social networking, together with the emerging capability of artificial intelligence to create content, has added a new dimension to the issue and significantly simplifies it, leading to the current"fake news" outbreak and data catastrophe.
It is apparent that individual fact-checkers working independently can't keep up with the sheer quantity of misinformation being shared daily. Several have therefore turned into innovative artificial intelligence for successful solutions to battle debatable content at scale, but that isn't without its challenges.
Linguistic cues like word patterns, syntax constructs, and readability attributes will need to be modeled to discriminate between individual and machine-generated content. State-of-the-art all-natural language processing (NLP) techniques are necessary for representing documents and words to effectively catch the contextual significance of words.
What's more, knowledge charts and innovative graph NLP calculations are needed to better mimic the interplay between various areas of textual content and represent the underlying topics in the record on higher-level abstractions.
In the event of visual information, improvements in picture editing and movie manipulation programs have made it considerably easier to create bogus videos and imagery. But, automated identification of exploited visual material at scale is hard and computationally costly. It involves cutting-edge compute infrastructure and execution of advanced computer vision, speech recognition, and multimedia evaluation to model the visual artifacts in different levels to comprehend a lot of facets like pixel and area amount inconsistencies, plagiarism, splicing, and spectrogram analytics.
Additionally, the prevalence of generative adversarial networks (GANs), as well as the high availability of resources that execute them have accelerated efforts to greatly generate deceptive multimedia which imitates the physiological and verbal activities of humans.
Countering deceptive multimedia creation and spread demands advanced AI models which are capable of artificial multimedia detection in addition to generation. The self-learning facet of the kind of AI, through constant re-training, necessitates enormous scale multimedia and cutting-edge computing ability to enhance the automatic alternatives for visual content comprehension and confirmation.
But, important recent improvements are made which can relieve some of those challenges.
Advances in large data sampling and processing provide smart and dependable strategies to pull smaller, nevertheless, agent data samples that encircle each of the essential routines and signs needed for the AI to extract strong insights, however with much reduced computational requirements.
Model compression and comprehension distillation plans have revealed the AI model sophistication, size, and inference prices may also be considerably reduced whilst keeping the identical amount of precision as the initial version.
These discoveries, together with machine learning methods like few-shot learning, have significantly reduced the compute engine prices on cloud infrastructures, thus making AI-based large data analytics economical for solving real-world issues like misinformation.
What's more, it's currently feasible to construct and maintain innovative ensemble AI systems that ingest and procedure unlimited amounts of information flows to extract actionable information regarding information source credibility, content authenticity, data veracity, social networking analysis of disinformation, its reach, and the influencers supporting it.
But, AI by itself can only do this much. The most precise AI versions can only be preserved through training and reinforcement by individual intellect and experience. And while AI is dependable to extract innovative insights regarding misinformation, it ought to be paired with individual analysts and domain experts -human-in-the-loop-AI -- to change the insights to be extremely interpretable and technical.
Additionally, mitigating the dangers and damages resulting from the viral spread of disinformation demands enforcement of timely, proactive countermeasures like dissemination of credible, confirmed information and analytic reporting into various characteristics of a disinformation story e.g. key actors and effort roots. This is only possible with prolonged (human + AI) intellect that may optimally exploit the energy of large info, human-in-the-loop AI, and advanced computing.
People and AI are both accountable for the issue of misinformation. To resolve it, we will need to alter human behavior to match our new functions as big-information customers. We will need to understand the significance of data credibility together with our data requirements. This is a slow process but until then, AI may decrease the dangers and work as a catalyst for change.
Relevant Courses You May Be Interested In :
AWS Technical Essentials Training